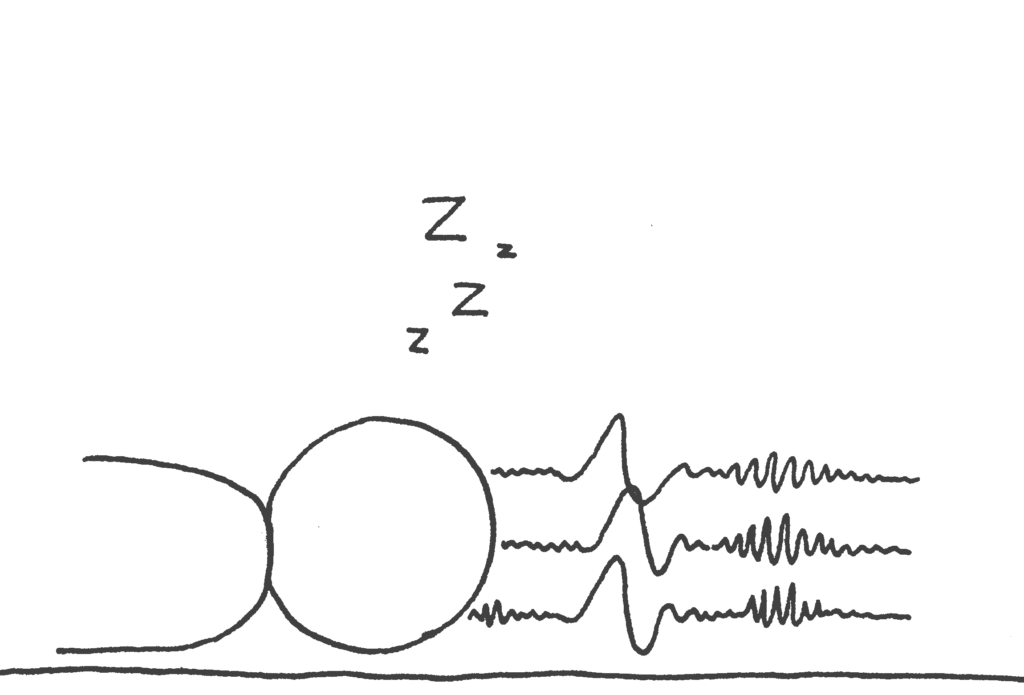
Sleep and Its Function
One part of the answer to the question why we sleep can be found in our memory. Because the brain is busy encoding new information continuously when we are awake, we need periods of sleep during which our brain can decide which memories to keep. In our lab, we investigate which memories are selected by sleep, how these are chosen, and which neuronal mechanisms are involved in this process.
We have found previously that many different types of memory benefit from sleep1: learning language2, learning sequences of movements3 and learning visual stimulus detection4, 5 all profit from sleep. There are mainly two types of mechanisms that affect memory during sleep, which are called “synaptic consolidation” and “systems consolidation”. While the former strengthens those synapses between neurons that have been directly involved in learning, the latter reorganizes memories across the brain.
Synaptic consolidation probably benefits from the specific neuromodulator environment present during sleep, e.g. low levels of acetylcholine6-8 and a generally reduced noradrenergic activity9. Sleep electrophysiology might contribute to synaptic or systems consolidation. We were the first to show that sleep spindles play a functional role for memory.10 Spindles could contribute to synaptic plasticity, but could also coordinate the hippocampal-neocortical dialogue. Slow oscillatory activity is another distinctive feature of sleep that we found to be implicated in memory processing, perhaps through its organizing function on other brain oscillations.11, 12
To allow systems consolidation, sleep spontaneously reprocesses freshly acquired memories – a process that we have recently shown in human electroencephalography (EEG) using a machine learning approach.13 Blocking synaptic glutamatergic transmission during sleep, required for this memory reactivation, can impair memory14, facilitating glutamatergic activity can enhance memory15. Memory reactivation can also be induced with external odour16 or sound cues17 during sleep and then strengthen certain memories over others. Central questions that we will try to answer in future studies are how reactivation in sleep changes memories and by which mechanisms reactivation affects synaptic and systems consolidation.
It is often suggested that sleep not only strengthens new memories, but that reprocessing also allows creative solving of problems during sleep. In a series of experiments, we could, however, not find any evidence for a problem-solving capacity of sleep.18, 19
Tracing the Memory Engram
The idea of systems consolidation directly entails that the location of a memory in the brain changes shifts over this process. While we found sleep-induced changes in brain activity compatible with this view20, we were intrigued by the observation that recall of a declarative, “hippocampus-dependent” word association task did not per se activate the hippocampus in functional imaging data. In follow-up studies, we found that the relative contribution of the hippocampus and an area of the posterior parietal cortex – the precuneus – show rapid inverse developments over learning within one single session: while the hippocampus disengages over learning repetitions, the precuneus becomes more and more involved.21
This latter area actually fulfils all criteria of a memory representation: it shows enduring changes in activity that relate to a specific memory, that can lie dormant for longer periods, and that are required for successful memory recall. Most recently, we were also able to show that these changes in the precuneus actually alter the brain’s microstructure in a way that can be detected in diffusion-weighted magnetic resonance imaging (dwMRI).22 The neocortex therefore proves to be a fast learner as well, a function that was previously only ascribed to the hippocampus. Such a neocortical memory, which can also develop through an implicit learning strategy called fast mapping, seems not to require sleep-related consolidation.23 We might therefore suspect that sleep and associated reactivation process mainly benefit memory that has not yet been fully integrated into neocortical networks. It remains open for further study and discussion whether the posterior parietal memory trace develops through rapid systems consolidation or whether it represents a second, parallel memory trace.
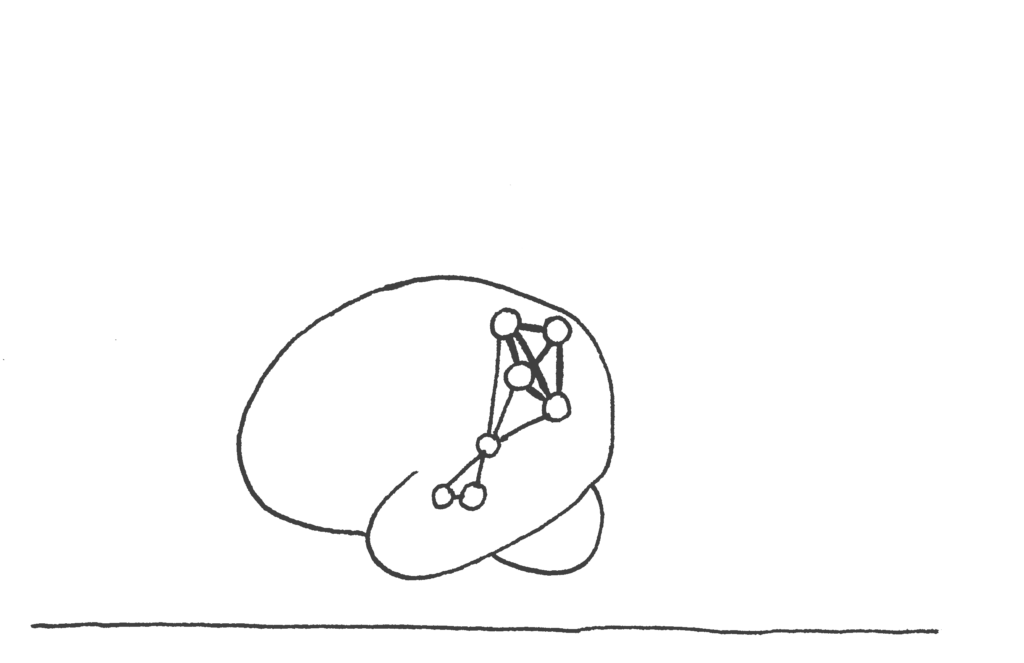
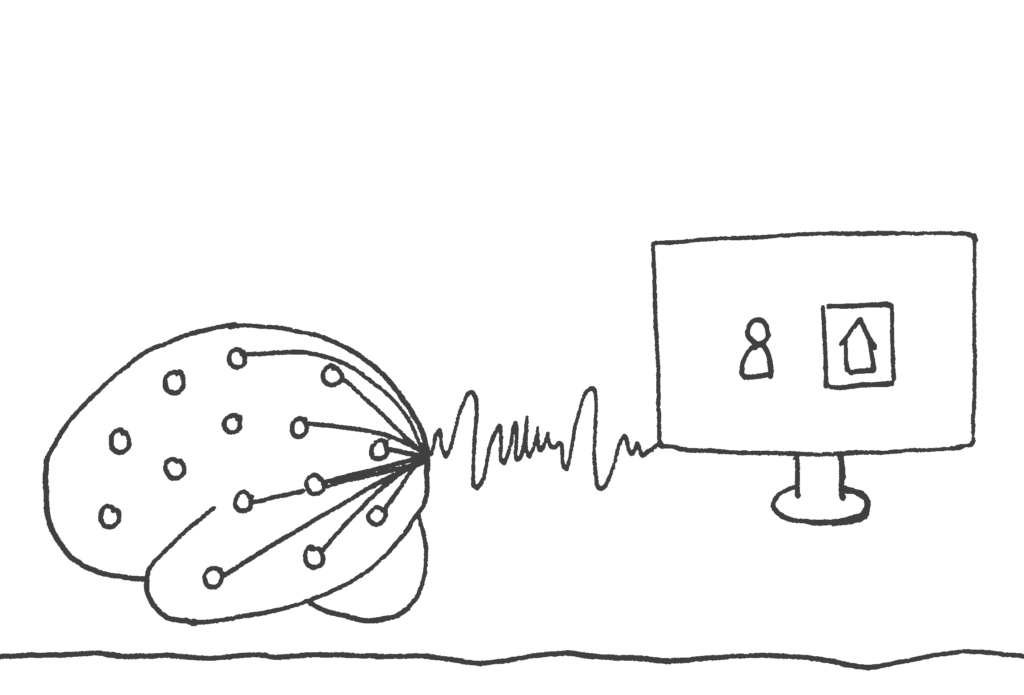
Applying Machine Learning to Hypothesis Testing
To investigate memory reactivation during sleep, we have to deal with very small signals that are hidden in huge sets of neurophysiological data, like all night 128-channel EEG recordings, MEG recordings and functional magnetic resonance imaging (fMRI) data. Therefore, we have started to use multivariate machine learning algorithms, in particular support vector machines, to find effects that are hidden to classical statistical approaches.13 However, it soon became obvious that the results of machine learning classification do not follow the normal distributions that we are used to see in classical analyses and that we depend on for hypothesis testing. Classification results can have strongly skewed distributions and also otherwise do not always behave as expexted.24 In particular, it is important to assure that any inherent structure in a data set, which would average out in classical analyses, does not affect classification, or that its effects are accounted for appropriately by the analysis.25 On the other hand, machine learning allows distinguishing the contributions of different aspects of a task to an outcome in ways that would not be possible in classical analyses.26 Obviously, the field of machine learning is rapidly growing in many directions. In future studies, we hope to make use of this progress, e.g. in the area of deep learning, and to develop more methods that are particularly suited to the needs of hypothesis testing in different experimental designs.

- Schönauer M, Pawlizki A, Köck C, Gais S (2014) Exploring the effect of sleep and reduced interference on different forms of declarative memory. Sleep. 37:1995-2007.
- Gais S, Lucas B, Born J (2006) Sleep after learning aids memory recall. Learn Mem. 13:259-262.
- Schönauer M, Grätsch M, Gais S (2015) Evidence for two distinct sleep-related long-term memory consolidation processes. Cortex. 63:68-78.
- Gais S, Plihal W, Wagner U, Born J (2000) Early sleep triggers memory for early visual discrimination skills. Nat Neurosci. 3:1335-1339.
- Geyer T, Mueller HJ, Assumpcao L, Gais S (2013) Sleep-effects on implicit and explicit memory in repeated visual search. PLoS ONE. 8:e69953.
- Gais S, Born J (2004) Low acetylcholine during slow-wave sleep is critical for declarative memory consolidation. Proc Natl Acad Sci U S A. 101:2140-4.
- Rasch B, Gais S, Born J (2009) Impaired off-line consolidation of motor memories after combined blockade of cholinergic receptors during REM sleep-rich sleep. Neuropsychopharmacology. 34:1843-53.
- Rasch BH, Born J, Gais S (2006) Combined blockade of cholinergic receptors shifts the brain from stimulus encoding to memory consolidation. J Cogn Neurosci. 18:793-802.
- Gais S, Rasch B, Dahmen JC, Sara S, Born J (2011) The memory function of noradrenergic activity in non-REM sleep. J Cogn Neurosci. 23:2582-92.
- Gais S, Mölle M, Helms K, Born J (2002) Learning-dependent increases in sleep spindle density. J Neurosci. 22:6830-6834.
- Mölle M, Marshall L, Gais S, Born J (2004) Learning increases human electroencephalographic coherence during subsequent slow sleep oscillations. Proc Natl Acad Sci U S A. 101:13963-8.
- Mölle M, Marshall L, Gais S, Born J (2002) Grouping of spindle activity during slow oscillations in human non-rapid eye movement sleep. J Neurosci. 22:10941-7.
- Schönauer M, Alizadeh S, Jamalabadi H, Abraham A, Pawlizki A, Gais S (2017) Decoding material-specific memory reprocessing during sleep in humans. Nat Commun. 8:15404.
- Gais S, Rasch B, Wagner U, Born J (2008) Visual-procedural memory consolidation during sleep blocked by glutamatergic receptor antagonists. J Neurosci. 28:5513-8.
- Feld GB, Lange T, Gais S, Born J (2013) Sleep-dependent declarative memory consolidation–unaffected after blocking NMDA or AMPA receptors but enhanced by NMDA coagonist D-cycloserine. Neuropsychopharmacology. 38:2688-97.
- Rasch B, Büchel C, Gais S, Born J (2007) Odor cues during slow-wave sleep prompt declarative memory consolidation. Science. 315:1426-9.
- Schönauer M, Geisler T, Gais S (2014) Strengthening procedural memories by reactivation in sleep. J Cogn Neurosci. 26:143-53.
- Schonauer M, Brodt S, Pohlchen D, Bressmer A, Danek AH, Gais S (2018) Sleep Does Not Promote Solving Classical Insight Problems and Magic Tricks. Front Hum Neurosci. 12:72.
- Brodt S, Pohlchen D, Taumer E, Gais S, Schonauer M (2018) Incubation, not sleep, aids problem-solving. Sleep. 41.
- Gais S, Albouy G, Boly M, Dang-Vu TT, Darsaud A, Desseilles M, Rauchs G, Schabus M, Sterpenich V, Vandewalle G, Maquet P, Peigneux P (2007) Sleep transforms the cerebral trace of declarative memories. Proc Natl Acad Sci USA. 104:18778-83.
- Brodt S, Pöhlchen D, Flanagin VL, Glasauer S, Gais S, Schönauer M (2016) Rapid and independent memory formation in the parietal cortex. Proc Natl Acad Sci USA. 113:13251-13256.
- Brodt S, Gais S, Beck J, Erb M, Scheffler K, Schönauer M (2018) Fast Track to the Neocortex: A Memory Engram in Posterior Parietal Cortex. Science. 362:1045-1048.
- Himmer L, Muller E, Gais S, Schonauer M (2017) Sleep-mediated memory consolidation depends on the level of integration at encoding. Neurobiol Learn Mem. 137:101-106.
- Jamalabadi H, Alizadeh S, Schönauer M, Leibold C, Gais S (2016) Classification based hypothesis testing in neuroscience: Below-chance level classification rates and overlooked statistical properties of linear parametric classifiers. Hum Brain Mapp. 37:1842-55.
- Jamalabadi H, Alizadeh S, Schonauer M, Leibold C, Gais S (2018) Multivariate classification of neuroimaging data with nested subclasses: Biased accuracy and implications for hypothesis testing. PLoS Comput Biol. 14:e1006486.
- Alizadeh S, Jamalabadi H, Schonauer M, Leibold C, Gais S (2017) Decoding cognitive concepts from neuroimaging data using multivariate pattern analysis. Neuroimage. 159:449-458.